ECSS Program Accelerates XSEDE User's Career in Geoinformatics

XSEDE ECSS user Tyson Swetnam created this model of global insolation over 365 days. Credit: Tyson Swetnam
While working as a wildland firefighter in the mid-2000s, Tyson Swetnam had no idea that he would eventually be led to a faculty position working with cyberinfrastructure. Swetnam, a research assistant professor at the University of Arizona, recently sat down with Robert Sinkovits, co-director of XSEDE's Extended Collaborative Support Services (ECSS) to discuss how the service played an important and large role in his career path and continued success in the area of geoinformatics.
Sinkovits: How did you first hear about the ECSS program?
Swetnam: I was a post-doctoral researcher with the Critical Zone Observatories project in 2014 working with a thermodynamic free
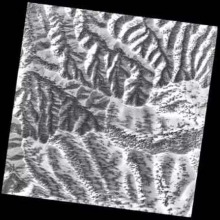
energy model that my collaborators had developed. I'd met a couple of folks from the iPlant Collaborative (now CyVerse) at University of Arizona who brought me on as a ‘client' in their fall course on cyberinfrastructure applications. The students' semester-long project became about helping me with my big data problem working with aerial Lidar and to calculate these models. With the help of the students, in one semester we created a distributed workflow on our campus' local high-performance computer, but we needed more.
My post-doctoral advisor, Jon Pelletier, saw some promise in the tool and applied for an XSEDE ECSS allocation. At the time, I hadn't heard of XSEDE or its ECSS program. When we got the award I was really excited, but also felt intimidated by these programmers whose ability to work with these big distributed systems was so impressive and so outside of my own skill set.
Before that class experience I'd never worked in Linux or on the command line, so I relied heavily on XSEDE's ECSS for guidance. I was specifically working with Yan Liu, a senior research programmer at the University of Illinois, and Mats Rynge, a computer scientist at the Information Sciences Institute at the University of Southern California. Mats and Yan got me up and running on XSEDE machines and guided me through the whole process. They helped me set up version control and containerized the workflow which made it reproducible. With their guidance and support I eventually became self-sufficient. Their patience and willingness to help teach me these new skills is something I am deeply grateful for.
Sinkovits: What specifically did you work on with the ECSS team?
Swetnam: We put together a pipeline that can be run anywhere (i.e. your laptop, cloud, or HPC) and depends on free and open source software for geographic information systems (GIS) and a workflow engine. We can calculate solar irradiation of any resolution surface model for every day (or even the hour) of the year. Those input solar energy layers establish the basis for our thermodynamic models. We then worked with the folks at OpenTopography.org, which is housed at the San Diego Supercomputer Center (SDSC), to host the tool for anyone to use. OpenTopo allows the users to select any location on the terrestrial earth surface and to calculate irradiation and hours of sun from global digital elevation models. The jobs from OpenTopography are run on the Open Science Grid (an XSEDE partner), coordinated by CyVerse virtual machines.
Sinkovits: Was your experience with ECSS worthwhile?
Swetnam: Yes! Without ECSS, this work would not have been possible. Indirectly, I learned more about how to use Linux, work on distributed computers via the command line, version control systems, and how to make my own containers. The results of which are an efficient, portable, scalable workflow that is deployed on OpenTopo, and can be downloaded from GitHub and reused by anyone.
Sinkovits: How did this impact your overall career?
Swetnam: My experience with the ECSS project led to a job offer from CyVerse in 2016, and thereafter my appointment to a research faculty position at the BIO5 Institute this spring.