CyVerse-Powered Workshop Teaches Hundreds of UNC Students Data Science Analysis
Approximately 350 UNC undergrad workshop students learned data science analysis using Jupyter notebooks powered by CyVerse.
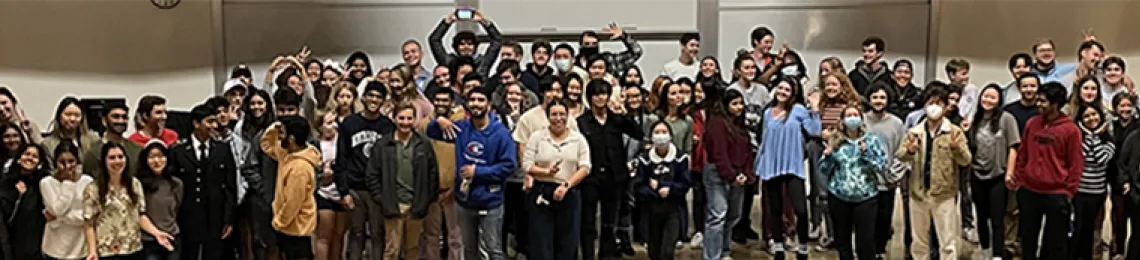
Last fall, approximately 350 undergraduate students at the University of North Carolina at Chapel Hill (UNC) used CyVerse to simultaneously launch Jupyter notebooks together as part of a core biochemistry course.
The course utilizes a synthetic approach where students are guided in the construction of community-led, reproducible workflows and are provided with Jupyter notebooks to facilitate learning through 3D imaging and hands-on experience. The interactive material was the brain-child of Elizabeth Brunk, an Assistant Professor jointly appointed in the departments of Pharmacology and Chemistry at the UNC Integrative Program for Biological & Genome Sciences. Many of the workflows used in the undergraduate course were developed by her team, including graduate students Kriti Shukla and Yue Wang.
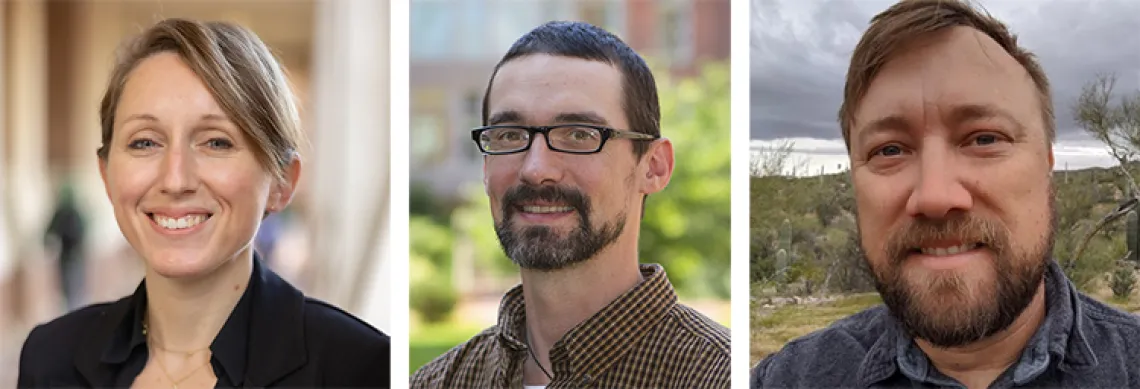
Left to right: Elizabeth Brunk (UNC), Brian P. Hogan (UNC), Tyson L. Swetnam (CyVerse)
The course is part of an effort by Brunk to expand data science education at UNC and to allow students from non-chemistry majors to translate big data into knowledge. In 2021, Brunk secured $65K in internal funding to provide a space for development of data analysis resources for graduate and undergraduate students. This space, termed the "Sci-Omics Lab," allows students who are pursuing data-driven experimental research to gain data science skills by analyzing data they generate themselves. It also engages computer science students to learn and understand from their peers in solving real-world biological issues.
Tyson L. Swetnam, Assistant Research Professor in Geoinformatics and Co-PI and Science Lead at CyVerse, explained how CyVerse was vital to the process. "CyVerse's Discovery Environment is scalable and allows each student to run larger virtual machines with more RAM and storage space than other free resources like MyBinder or Google Colab, which are both excellent for teaching," Swetnam said. "By enrolling the students with Basic (free) accounts through our workshop portal, we were able to grant everyone access to the same containerized JupyterLab with the 3D visualization notebooks pre-loaded."
Brunk teaches a large enrollment biochemistry course along with Brian P. Hogan, a Teaching Professor in the UNC department of Chemistry. Hogan, who has taught the chemistry class for 20 years, explains that the Intro to Biochemistry course is a junior/senior level course taken by biology and chemistry majors as well as pre-med majors. "UNC redesigned the curriculum to stress the importance of authentic research. This class gives students an opportunity to do real research and develop research skills. That's important for us as an institution," said Hogan.
"In the past, we have polled students in our workshops, and we consistently saw that 4 out of 5 students wished they had some exposure to coding or omics data as undergrads, so this course helps fill that gap," Brunk said. "It's a different way for them to contextualize what they are learning. In the first part of the class, they learn about protein structure, and with a Jupyter notebook, they can use Python-based libraries that enable visualization of three-dimensional protein structures. Using this approach, students can have a more experiential experience with the course material related to protein structure and function. Jupyter notebooks are a groundbreaking tool that effectively lowers the barrier of entry for students who have never coded in Python before. It's important to note that both Brian and I have been happily surprised with the number of students who learn that they genuinely enjoy coding and apply the skills they gain from this class in their undergraduate research experiences."
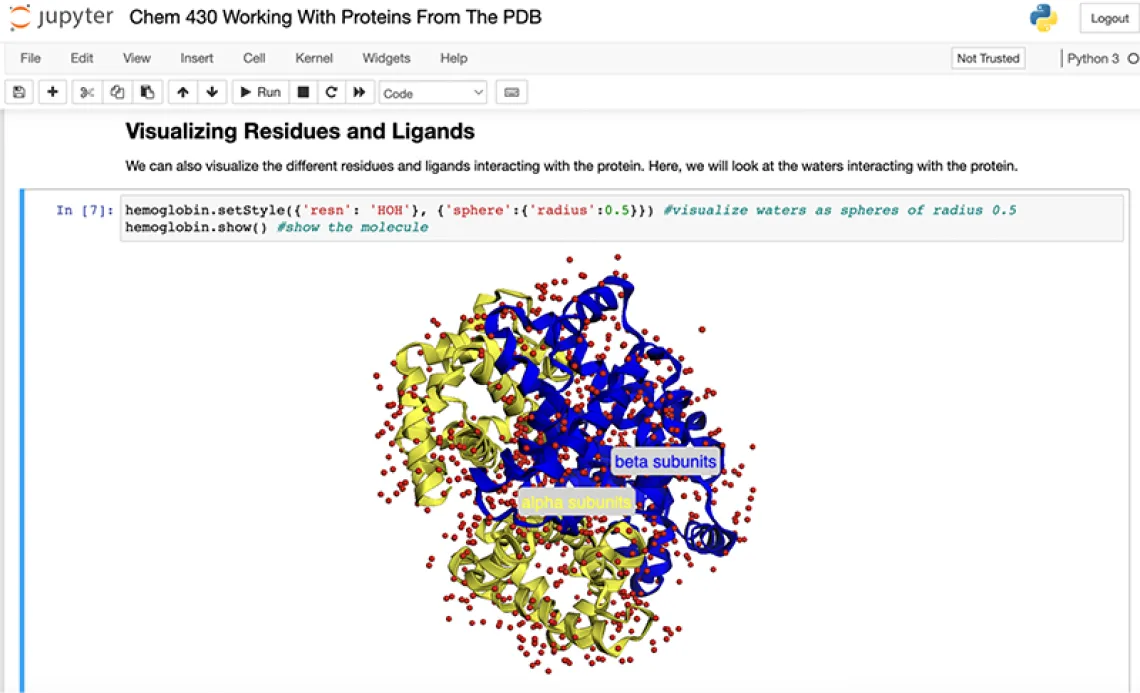
Plant protein image from a Jupyter notebook (Credit: Elizabeth Brunk)
As Brunk sees it, one of the main challenges for big data analysis is the intellectual divide between experimental scientists who produce data and the computational scientists who analyze the data. Often, these two sides may not speak the same language, and it can be difficult to maximize the gain of big data that is being generated or already generated. As both sides are important to moving big data science forward, Brunk believes it is vital to train future scientists earlier in their careers in order to bridge this gap and encourage both sides to work together more effectively.
The Sci-Omics Lab serves as a space where students in experimental omics fields can learn standard data analysis methods and work with others to utilize those methods with their own data. Graduate students with backgrounds in computational analysis of biological data have led workshops to train other students through workflows developed by members of the lab. The workshops are designed to ensure that students are taught through a synthetic approach to ensure they understand the required steps and that they see the code, create a strategy, and make informed decisions about the process. In the future, students will be able to sign up for times to bring their own data for analysis to the lab.

Full class picture (Credit: Elizabeth Brunk)
"There is a severe deficiency of people who can understand both the biology and coding side of big data analysis," Brunk said. "It may be that graduate school is too late to start training students who want to bridge these aspects of big biological data. If given exposure at the undergraduate level, students can have new opportunities to decide whether it is something they enjoy and that motivates them. We have numerous examples of students who loved this experience, and that's what makes it all worthwhile."